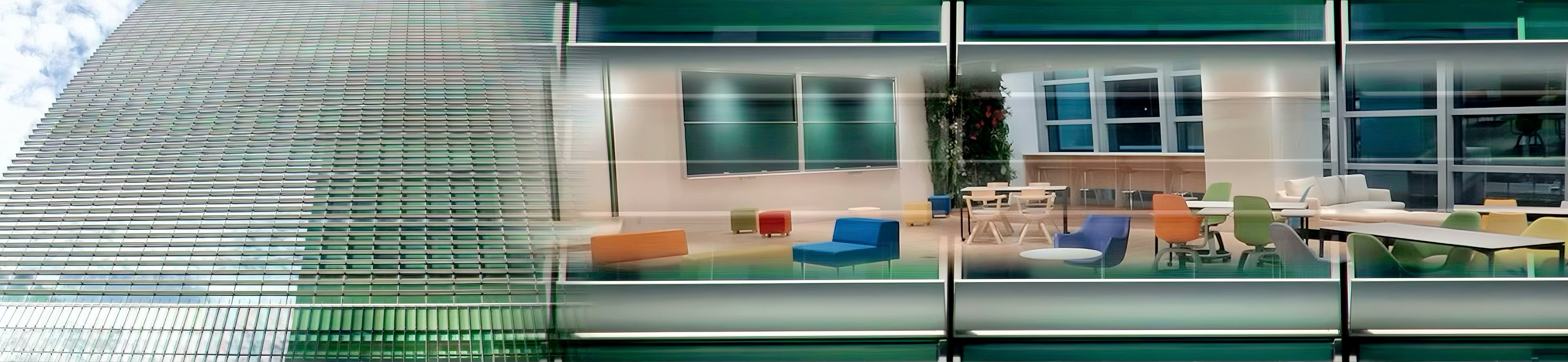
Our team is affiliated with the RIKEN Center for Advanced Intelligence Project (AIP), located in Tokyo. You can also visit our official pages on the RIKEN website and AIP website.
Research
Modern machine learning technologies often demand vast amounts of data, large-scale models, and considerable computational resources, which also results in challenges related to the reliability and interpretability of well-trained models. Our team aims to develop efficient, robust, and interpretable machine learning models and algorithms, along with their theoretical analysis. We focus on several key directions including tensor methods for machine learning, robust and interpretable machine learning and quantum machine learning. Our research spans various areas such as self-supervised learning, unsupervised representation learning, multi-modal learning and deep generative models. We also seek collaborations in practical domains such as brain-computer interfaces, medical diagnosis, and etc.
Research Topics
-
Tensor Methods for Machine Learning
Our research lies in the intersection of tensor networks and machine learning. We investigate tensor network theory with its algorithms and leverage tensor network representations to address critical challenges in deep learning, such as data and parameter efficiency, adversarial robustness and interpretability of deep generative models, as well as the theoretical understanding of tensor-based learning methods.
-
Trustworthy Machine Learning
Our research in trustworthy machine learning focuses on the robustness and interpretability. We aim to develop robust models that can handle noisy and incomplete data, as well as adversarial attacks, while mitigating potential performance degradation. In terms of interpretability, we intend to design inherently interpretable models and explore the relationship between robustness and interpretability.
-
Quantum Machine Learning
Our research focuses on quantum-inspired machine learning models and hybrid quantum-classical algorithms, aiming to harness quantum advantages to enhance machine learning technologies. We intend to investigate tensor networks for data encoding, quantum circuit compression, and quantum algorithms. This research is supported by the RIKEN Quantum project.
For more information, please visit our Publications and Events pages. We are actively seeking collaborations and welcome you to join us.
News
Our team has one paper accepted by NeurIPS 2024.
Sep 2024Our proposal for international workshop on CoLoRAI - Connecting Low-Rank Representations in Al in conjunction with AAAI 2025 has been accepted.[Link]
May 2024Our team has five papers accepted by ICML 2024.
Feb 2024Our team has one paper accepted by CVPR 2024.
Jan 2024Our proposal for international workshop on TMME: Tensor Models for Machine lEarning - Empowering Efficiency, lnterpretability, and Reliability in conjunction with IEEE CAI 2024 has been accepted.
Jan 2024Our team has two papers accepted by ICLR 2024 with one spotlight and one poster presentation.
For more past news, please visit News List